We publish many datasets alongside our papers, and encourage others to use them. We apply flexible open licensing where we can, but note that some datasets contain third party restrictions. Please find links and descriptions below, and get in contact if you’d like to find out more about these.
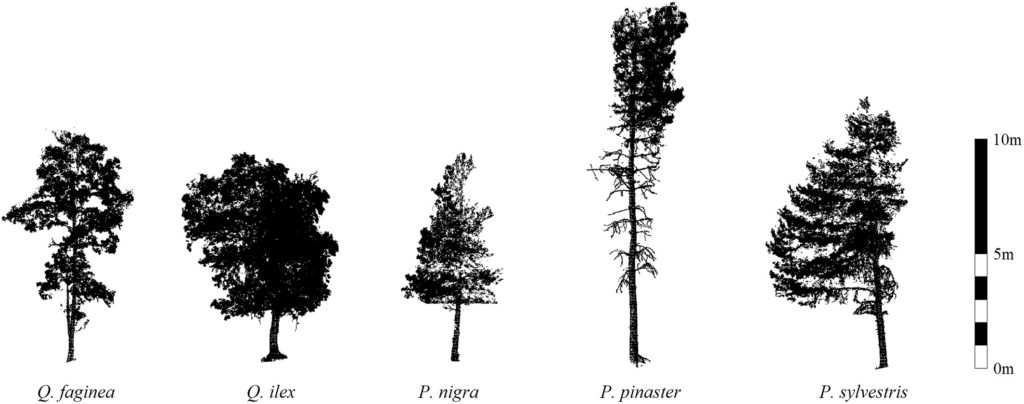
Individual TLS tree clouds collected from both Alto Tajo and Cuellar in Spain. Owen et al. (2022)
c. 2500 individual TLS-scanned tree point clouds, with species tags. Used in Owen et al. (2021), Allen et al. (2022) and Flynn et al. (2023).
36 30 x 30 m plots across two sites in Spain were scanned using a Leica HDS6200 scanner (3.2mm resolution at 10 m) following a grid pattern. Scans were co-registered and individual trees segmented using a combination of treeseg (Burt et al. 2019) and manual extraction/refinement. Trees in this database have been zero-centred and downsampled to 5 cm . Individual tree species ID (Quercus faginea, Quercus ilex, Pinus nigra, Pinus sylvestris and Pinus pinaster) and whether a tree is a multi-stem or single-stem are given in the metadata file.
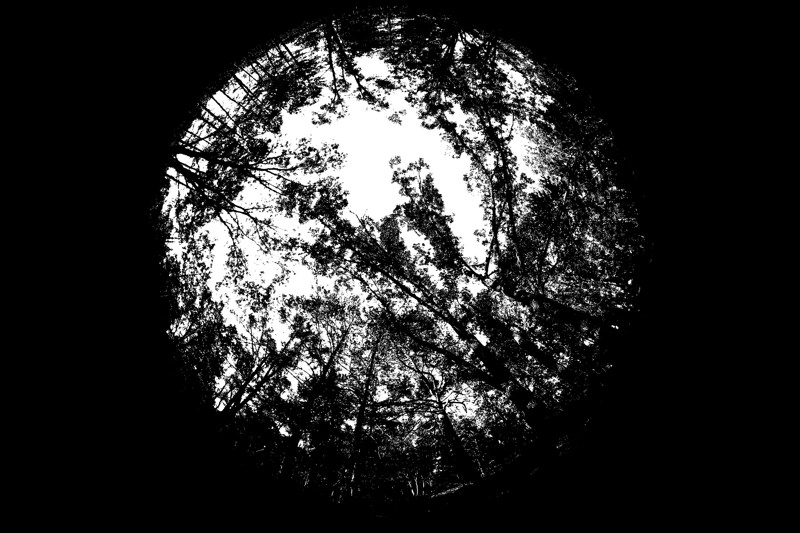
DHP images collected from Alto Tajo and Cuellar in Spain. Flynn et al. (2023).
Digital Hemispherical Photography images taken in 33 30 x 30 m plots across two sites in Spain, collected at the same time as Owen et al. 2022. Used in Flynn et al., 2023, with accompanying code.
Images were taken on a 10 m grid, making 16 locations per plot. At each location, DHP images were captured with three exposure settings, levelling a Canon EOS 6D full frame DSLR sensor with a Sigma EX DG F3.5 fisheye lens. For each RGB image, the blue band was extracted, as this best represents sky/vegetation contrast. Automatic thresholding was carried out using the Ridler and Calvard method (1978), creating a binary image of sky and vegetation.
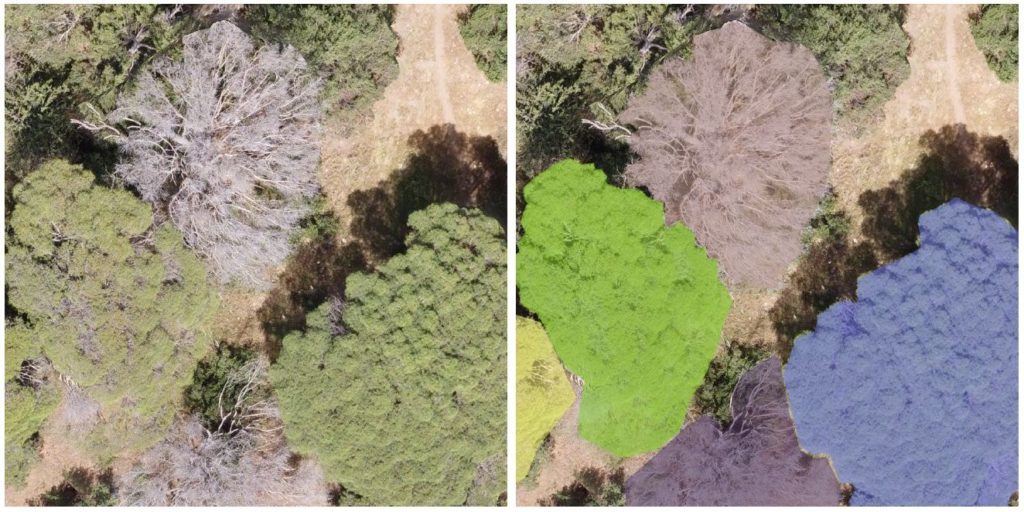
Almorox Crown Dataset – Crown Instance Segmentation Data & Dieback Estimates. Allen et al. (2024)
COCO, imagery & inventory data for manuscript “Low-Cost Tree Crown Dieback Estimation Using Deep Learning-Based Segmentation” (Allen et al., 2024, Environmental Data Science). Archive split into parts to aid uploading.
Drone imagery captured in Pinus pinea forests suffering drought-induced dieback in Pinar de Almorox, near Madrid, Spain. Imagery captured across c. 1500 ha of forest using a DJI Mavic Mini drone in May/June 2021, with orthomosaics generated using Agisoft Metashape.
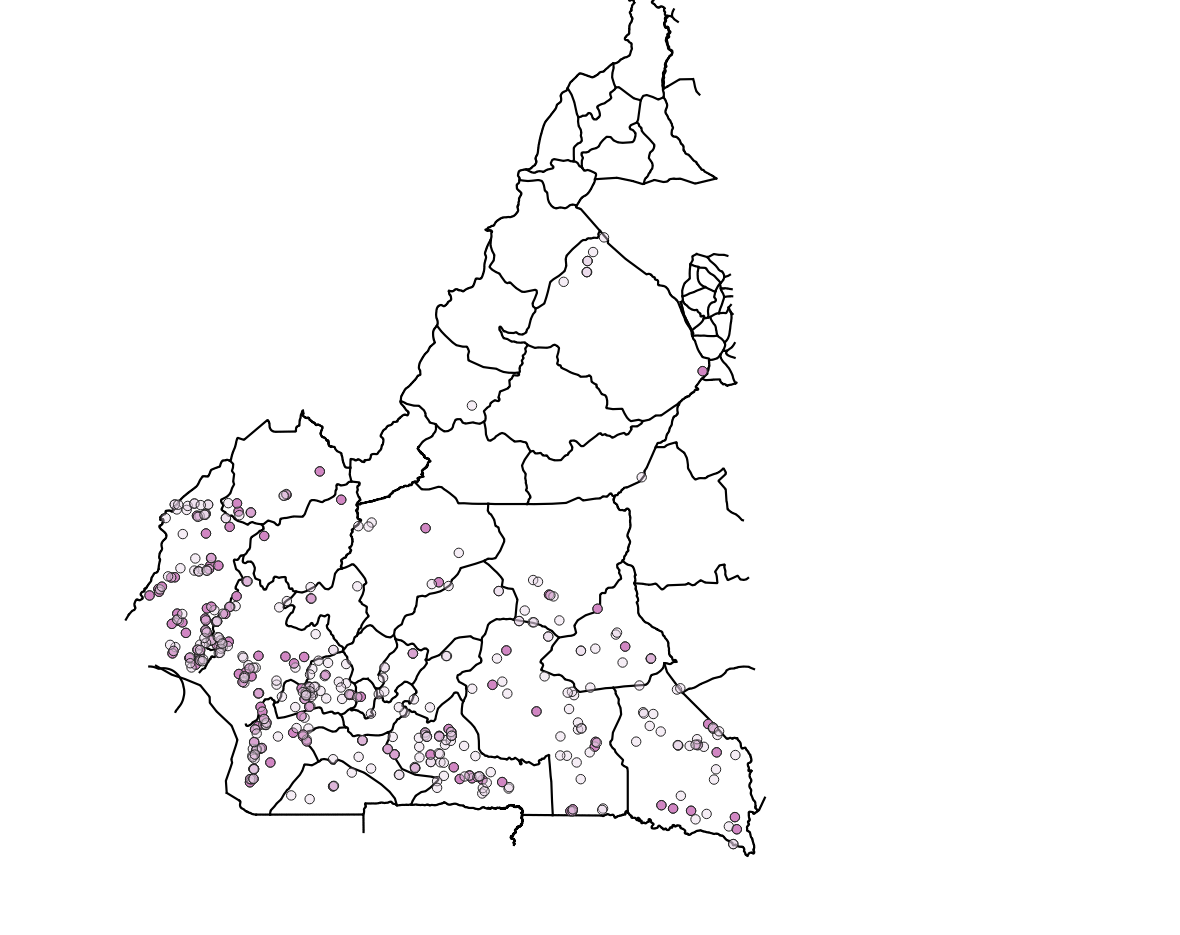
Labelled dataset to classify direct deforestation drivers in Cameroon. Debus et al. (2024).
Dataset used in Debus et al. (2024).
This dataset includes the images (visible bands for Landsat-8 or NICFI PlanetScope), auxiliary data (infrared, NCEP, forest gain, OpenStreetMap, SRTM, GFW), and data about forest loss (Global Forest Change) used to train, validate and test a model to classify direct deforestation drivers in Cameroon.